You train the model, taking the effects of quantization errors into account. This is used when post-training quantization techniques lead to worse performance.
Fake quantization-dequantization modules are added at points where quantization normally occurs (activations, weights and biases). These tensors are quantized and dequantized immediately.
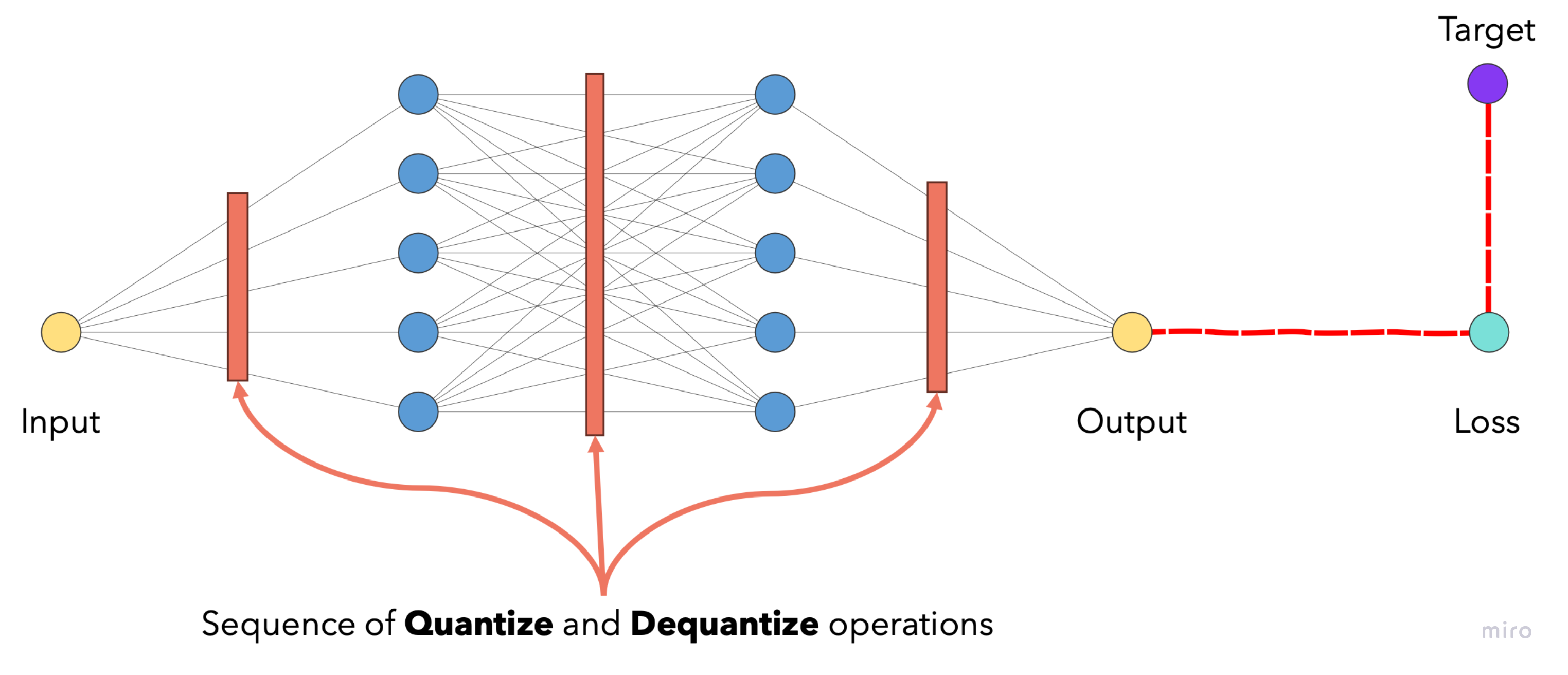
The result is that the model’s parameters have to be guided to a point on the loss function/surface where it is robust against quantization errors—leading to a model that performs well despite the effects of quantization
https://ar5iv.labs.arxiv.org/html/2004.09602
Some source mention fine-tuning a pre-trained model1 for a few more epochs while others2 say train it from scratch.